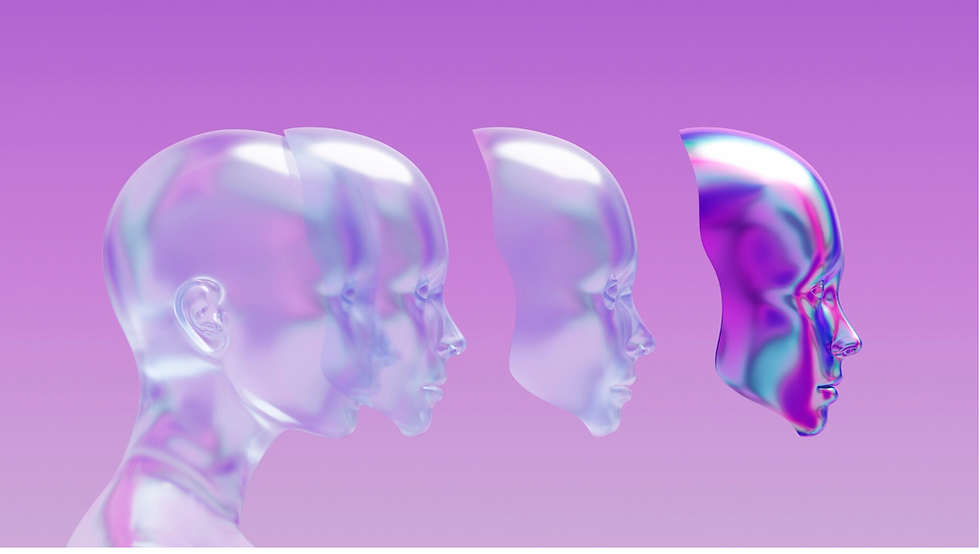
AI and algorithmic bias remains a complex and ongoing challenge for humans. According to wikipedia, Algorithmic bias describes systematic and repeatable errors in a computer system that creates “unfair" outcomes such as "privileging" one category over another - in ways different from the intended function of the algorithm. On another hand, Algorithmic bias can also occur when an algorithm produces results that are “systematically prejudiced” due to incorrect assumptions in the machine learning process or due to “already biased data”.
The quality and diversity of data directly impact outcomes since AI systems are often designed to learn from data. Bias can originate from limited data input, unfair algorithms, use of old data, lack of transparency about the data collection process and also from bias practices during AI development. Many factors can also lead to bias. This factors include: the design of the algorithm, the data collection process, the data selection process, the decisions relating to the way data is coded, the way the collected data is used as well as others. There can be various types of AI bias: model bias, data bias, prejudice in design, human bias, feedback loops, socio-technical factors and others.
A data collection and selection process that is not inclusive enough when used to train an AI system will produce biased outcomes. This becomes concerning if the AI systems is used in crucial areas like law enforcement, healthcare or criminal justice. The created bias can heighten existing inequalities and make it more difficult to address issues like racial or gender discrimination and can lead to systemic problems where certain groups become marginalised because of decisions made by such biased systems.
The adoption of Artificial intelligence in critical areas such as law enforcement, the justice system, hiring, healthcare system, finance as well as other day-to-day activities makes it imperative for leaders, organisations, data experts, machine learning experts and other professionals - to address the issue of algorithmic bias that comes with AI. It should be noted that even if the data is of good quality, the way the algorithm is designed (the model) can still lead to biased outcomes. This biased outcomes leaves humans with some questions that needs to be answered. Some of these questions include:
When algorithms make biased decisions, who is responsible? For example, If a biased AI system hires only from a particular race, origin or gender or if an AI system causes harm in healthcare — how will or how should the culprits be identified? Will the culprits be the developers who processed and worked with the data, the machine learning experts, the data providers or the institutions using the system?
How should this be addressed ethically or legally? Who takes the blame or pays for the damage caused ?
These are areas that humans must address and fine-tune before the full adoption of AI. The lack of accountability in these areas will lead to the loss of trust in the system and will further re-enact and heighten systemic problems that have almost previously been eradicated.
Organisations should recognise that there are key challenges that come with addressing bias. Questions such as how to strike the balance between making algorithms efficient and fair - should be deeply thought about. While answering these questions, it should be understood that the definition of “fairness” varies widely and what is fair for example in the context of healthcare may not be fair in the context of hiring. These differing perspective makes it difficult to develop a universal definition of “fairness” that applies across all domains. Organisations should embrace regular audit of AI systems to check for fairness and accountability, Set-up “AI ethics boards” to help identify whats fair and ethical, Invest early in “bias-detection tools” and raise continuous awareness amongst employees to help ensure the system is transparent and bias free.
Good collaboration between the public and private sectors to help define whats fair or not, increased public awareness and education, more academic research, inclusion of regulatory authorities that will ensure fairness in AI systems as well as corporate responsibility - all play vital roles in mitigating bias and ensuring that AI technologies are fair and ethical.
Need help with training your teams / leaders ?
Contact us : https://www.gritscales.com/contact
Timely writeup. Distruptive technology like GAI and all the various forked versions of LLMs are too powerful to be left in the hand of certain powerful individual or group. They should either be open-sourced or made not-for-profit, and heavily regulated to redress privacy, every form of bias (political and racial especially), ethical concerns, social injustice, infodemics, existential threats, liability, and systematic control of peoples mind and opinion. Source of training dataset needs to be independently verified for accuracy of information for example, but who does that.....genuine human concerns and fears presently outweighs the present benefits of these models, hence the need for further
This is excellent. Definitely a subject that everyone needs to be discussing